Section 4 Calibration and Validation
4.1 Dataset Split
To calibrate and validate the model, the observed dataset of presence/absence by catchment (see Observation Data) was randomly split using 80% of the catchments for calibration and 20% for validation.
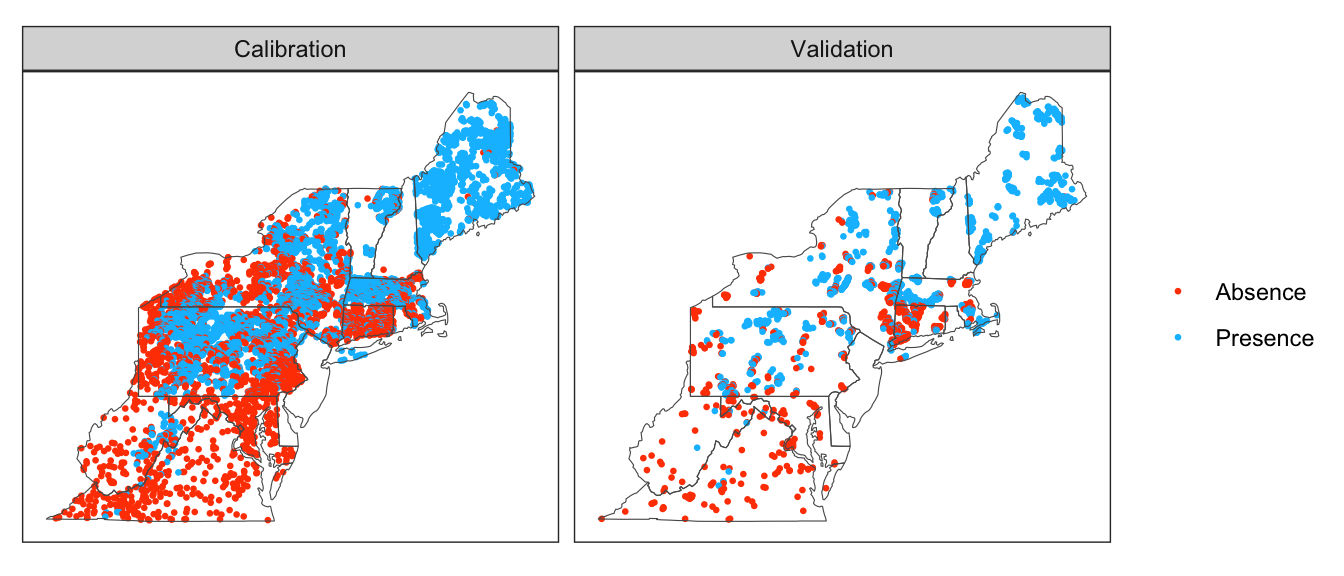
Figure 4.1: Calibration and Validation Splits
Partition | Presence | Absense | Total | % Presence |
---|---|---|---|---|
Calibration | 6,690 | 3,612 | 10,302 | 64.9% |
Validation | 1,822 | 1,017 | 2,839 | 64.2% |
Total | 8,512 | 4,629 | 13,141 | 64.8% |
4.2 Calibration
The following output summarizes the fitted model using the calibration subset.
Generalized linear mixed model fit by maximum likelihood (Laplace
Approximation) [glmerMod]
Family: binomial ( logit )
Formula: presence ~ mean_jul_temp + (1 | huc8)
Data: model_gm_data
Control: glmerControl(optimizer = "bobyqa")
AIC BIC logLik deviance df.resid
8513.1 8535.0 -4253.5 8507.1 10934
Scaled residuals:
Min 1Q Median 3Q Max
-17.9986 -0.3634 0.1320 0.4235 12.4987
Random effects:
Groups Name Variance Std.Dev.
huc8 (Intercept) 5.139 2.267
Number of obs: 10937, groups: huc8, 202
Fixed effects:
Estimate Std. Error z value Pr(>|z|)
(Intercept) 20.66823 0.69572 29.71 <2e-16 ***
mean_jul_temp -1.08706 0.03498 -31.08 <2e-16 ***
---
Signif. codes: 0 '***' 0.001 '**' 0.01 '*' 0.05 '.' 0.1 ' ' 1
Correlation of Fixed Effects:
(Intr)
mean_jl_tmp -0.966
The estimated fixed effect for mean July temp (mean_jul_temp
) was -1.09. Because the estimated value is negative, the occupancy probability is higher at lower stream temperatures. Figure @(fig:calib-fixed) contains a marginal effects plot showing the predicted probability over varying mean July stream temperatures (excluding random effects).
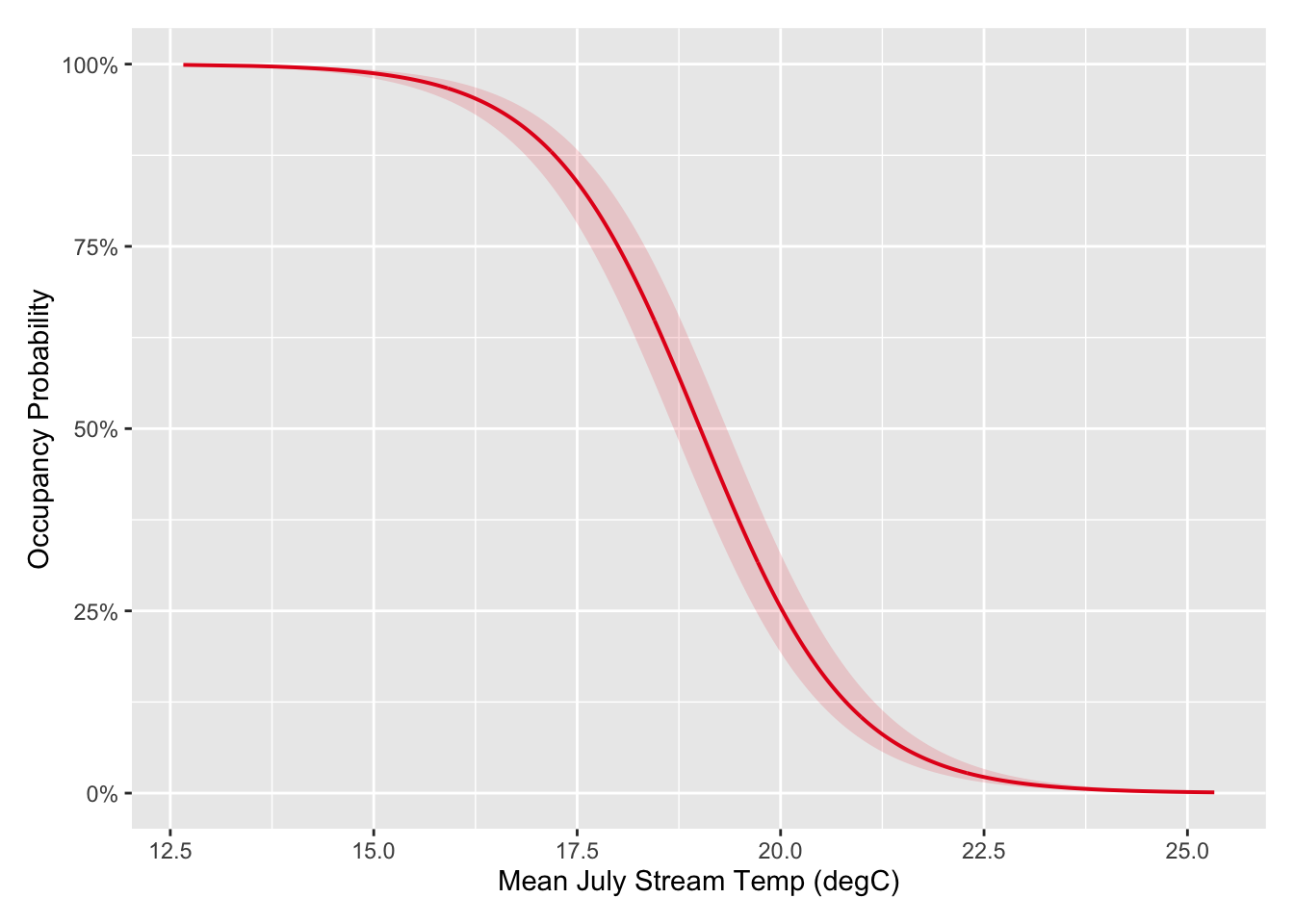
Figure 4.2: Marginal Effects Plot for Mean July Stream Temperature.
The random effect intercept varies by HUC8 basin. Basins with higher values tend to have higher occupancy probabilities for a given mean July stream temperature. Some HUC8 basins do not have an estimated value because there was no observations with the calibration dataset.
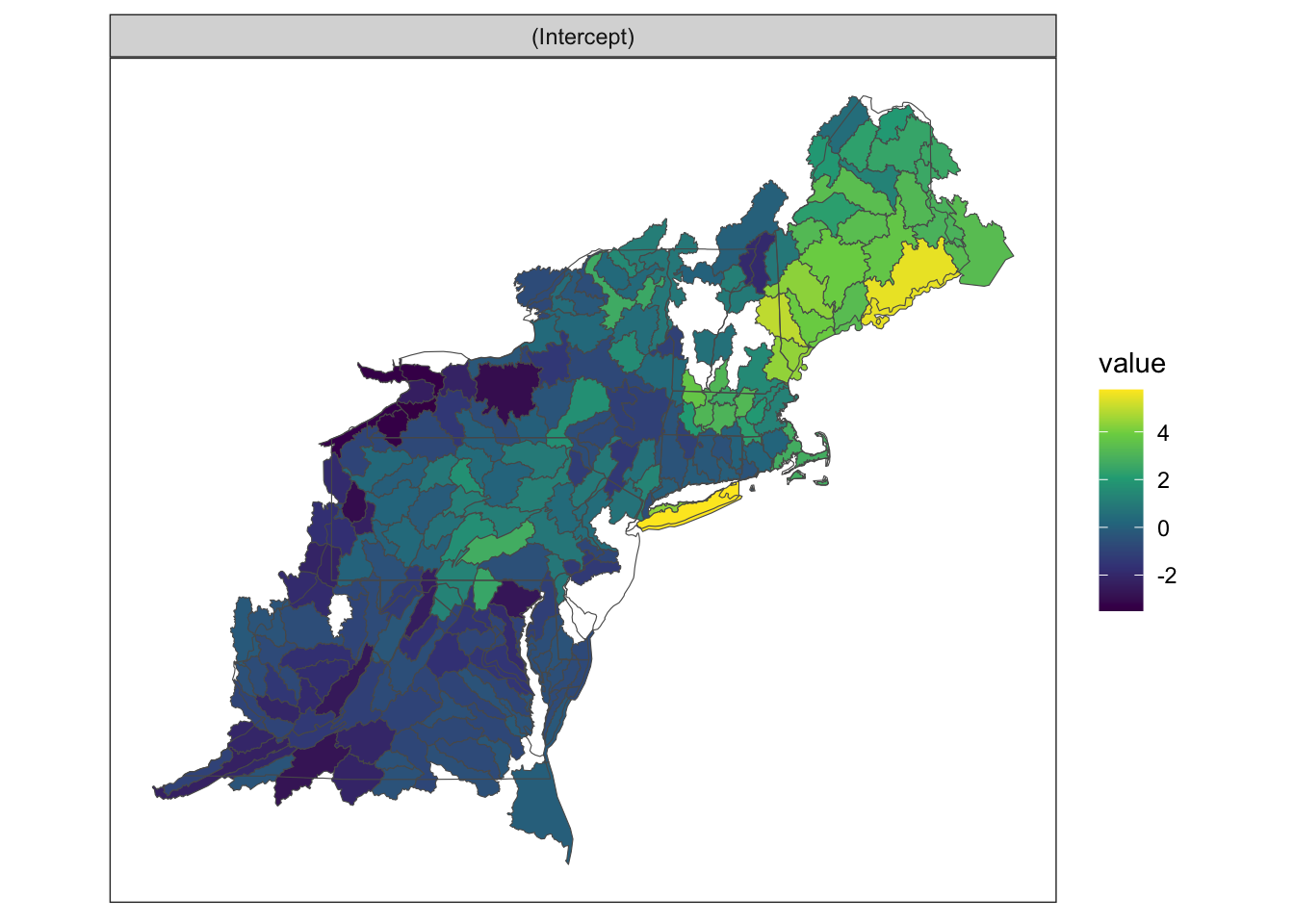
Figure 4.3: Random Effect Intercept by HUC8 Basin
The model accuracy and performance is summarized by a series of metrics computed from the confusion matrix, which contains the total number of true positives, true negatives, false positives, and false negatives. In the 2x2 table at the top of the following output, the columns (Reference
) refer to the observed condition in each catchment (1
= presence, 0
= absence), while the rows (Prediction
) refer to the predicted condition. The predicted probabilities were converted to presence/absence using a 50% cutoff. The remaining output provides a series of performance metrics computed from the confusion matrix using the confusionMatrix()
of the caret
package (Kuhn, 2022). See the help page for that function, as well as this Wikipedia article, for definitions of each metric.
Confusion Matrix and Statistics
Reference
Prediction 0 1
0 2766 720
1 1074 6377
Accuracy : 0.836
95% CI : (0.8289, 0.8429)
No Information Rate : 0.6489
P-Value [Acc > NIR] : < 2.2e-16
Kappa : 0.6322
Mcnemar's Test P-Value : < 2.2e-16
Sensitivity : 0.8985
Specificity : 0.7203
Pos Pred Value : 0.8559
Neg Pred Value : 0.7935
Prevalence : 0.6489
Detection Rate : 0.5831
Detection Prevalence : 0.6813
Balanced Accuracy : 0.8094
'Positive' Class : 1
4.3 Validation
Using the calibrated model, predicted probabilities were computed using the indendent validation dataset.
The confusion matrix for the validation dataset indicates slightly lower accuracy (0.84 vs 0.81), but overall comparable performance. These results suggest that the model does not suffer from overfitting.
Confusion Matrix and Statistics
Reference
Prediction 0 1
0 599 227
1 190 1188
Accuracy : 0.8108
95% CI : (0.7938, 0.827)
No Information Rate : 0.642
P-Value [Acc > NIR] : < 2e-16
Kappa : 0.5926
Mcnemar's Test P-Value : 0.07791
Sensitivity : 0.8396
Specificity : 0.7592
Pos Pred Value : 0.8621
Neg Pred Value : 0.7252
Prevalence : 0.6420
Detection Rate : 0.5390
Detection Prevalence : 0.6252
Balanced Accuracy : 0.7994
'Positive' Class : 1
The following table compares the performance metrics between the two subsets.
Metric | Calibration | Validation |
---|---|---|
Accuracy | 0.836 | 0.811 |
Sensitivity | 0.899 | 0.840 |
Specificity | 0.720 | 0.759 |
Pos Pred Value | 0.856 | 0.862 |
Neg Pred Value | 0.793 | 0.725 |
Precision | 0.856 | 0.862 |
Recall | 0.899 | 0.840 |
F1 | 0.877 | 0.851 |
Prevalence | 0.649 | 0.642 |
Detection Rate | 0.583 | 0.539 |
Detection Prevalence | 0.681 | 0.625 |
Balanced Accuracy | 0.809 | 0.799 |
Lastly, Receiver Operator Characteristic (ROC) curves and Area Under the Curve (AUC) values also shows comparable performance between the calibration and validation subsets.
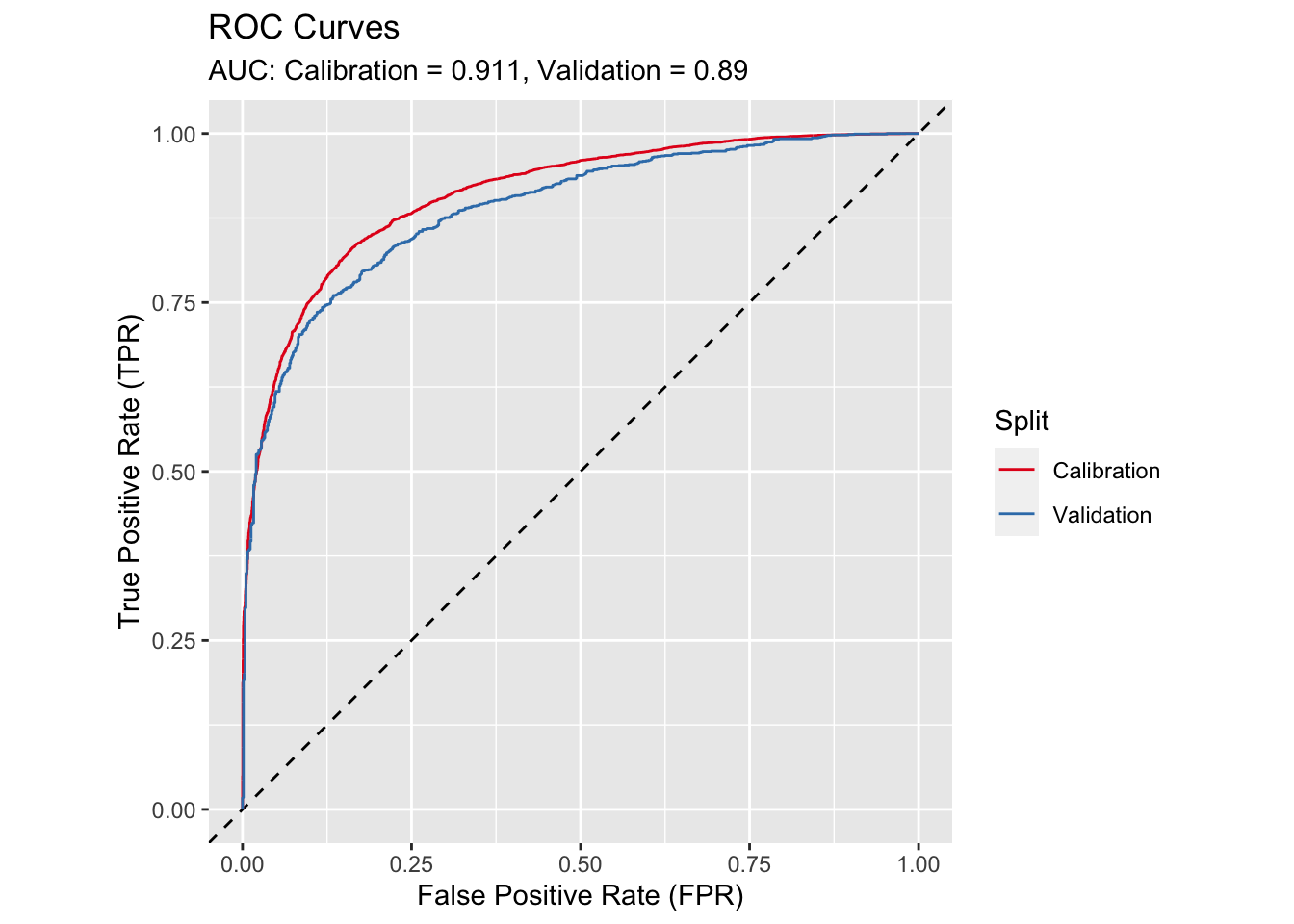
Figure 4.4: ROC Curves for Calibration and Validation